Tanh vs Sigmoid
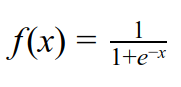
I've been exploring some very common activation functions and visualizing what effects these activation functions bring to the output at each layer. Before we dive into some details, let me first introduce these activation functions. I. Activation functions 1. Sigmoid Sigmoid is one of the most common activation functions. The output of sigmoid can be interpreted as "probability" since it squashes the values to the range (0,1) so it is very intuitive to use sigmoid at the last layer of a network (before classifying). Sigmoid function takes on the form Sigmoid is a smooth function and is differentiable; however it suffers from gradient saturation issue when x is very large or very small (i.e. the gradient is very small). As depicted in the figure below, when f approaches either 0 or 1, the gradient becomes very small. Another issue of the sigmoid function is that the output of it is not zero-centered. Therefore, in many cases tanh is preferred. 2. Tanh ...